After years of investment in marketing data analytics, many CMOs are frustrated and preparing to cut their losses. Gartner’s new research highlights the problem, but no one is talking about the obvious solution: it’s time to throw marketing analytics a lifeline.
Written by Alistair Adam, Head of Analytics at Optima Connect.
It’s sink or swim time for marketing data analytics.
That’s what I took from Gartner’s Marketing Data and Analytics Survey 2020: Optimism Persists as Results Fall Short of Expectations, at least.
One of the report’s key findings is that: “Despite CMOs prioritizing investments in marketing data and analytics, this year’s Marketing Data and Analytics survey finds just over half of senior marketing leaders disappointed in the results they receive from analytics investments thus far.”
And little wonder.
Incredibly, “nearly half (44%) of respondents in this year’s survey say they’re unable to measure marketing ROI.” This is despite marketing analytics departments growing by an average of 10 FTEs over the last two years. Data Science comes under particularly heavy fire from CMOs, taking top spot in the ‘disappointment list’ for failing to meet expectations. In a turn that may ring alarm bells for many marketing analytics teams, Gartner states that “by 2023, 60% of CMOs will slash the size of their marketing analytics departments in half because of failed promised improvements.”
Data Engineering: The Missing Link in Marketing Analytics?
In this article, I’ll reflect on how many of the most significant barriers facing analysts appear to relate to data quality, disconnected data sources, and the huge time drain associated with data management and data preparation.
As we’ve argued before, these are barriers that analysts themselves should not be tasked with resolving. Improving data quality, integrating data sources, preparing high volumes of complex data… these are all specialised data engineering tasks. As we’ve seen time and again at Optima Connect, the best return on investment in analytics and data science comes when marketing leaders also recognise the vital role and contributions of data engineers.
READ MORE – Data Science vs Data Engineering: Role Comparison (Part 1)
Although Gartner’s report does not explicitly reference data engineering as a distinct skillset, in our opinion the respondents’ answers to the survey repeatedly highlight the need for investment in data engineering capabilities in addition to data analysts and data scientists.
To support this assertion, first let’s look at what Gartner discovered when asking CMOs why they feel they’re not getting what they need from their marketing analytics teams.
Marketing Leaders’ #1 Problem: Poor Data Quality
Figure 4 of Gartner’s report (excerpt reproduced below), titled ‘Top Reasons Why Analytics Is Not Used in Informing Decisions’, reveals that CMOs consider poor data quality to be one of the biggest problems with their marketing analytics. We’ve written before about why poor quality data is the single biggest reason that data analytics projects fail. We believe Gartner’s survey supports this. As we argue in the linked article above, there is simply no better way to undermine the value of your data analytics team than to expect them to perform miracles with terrible data.
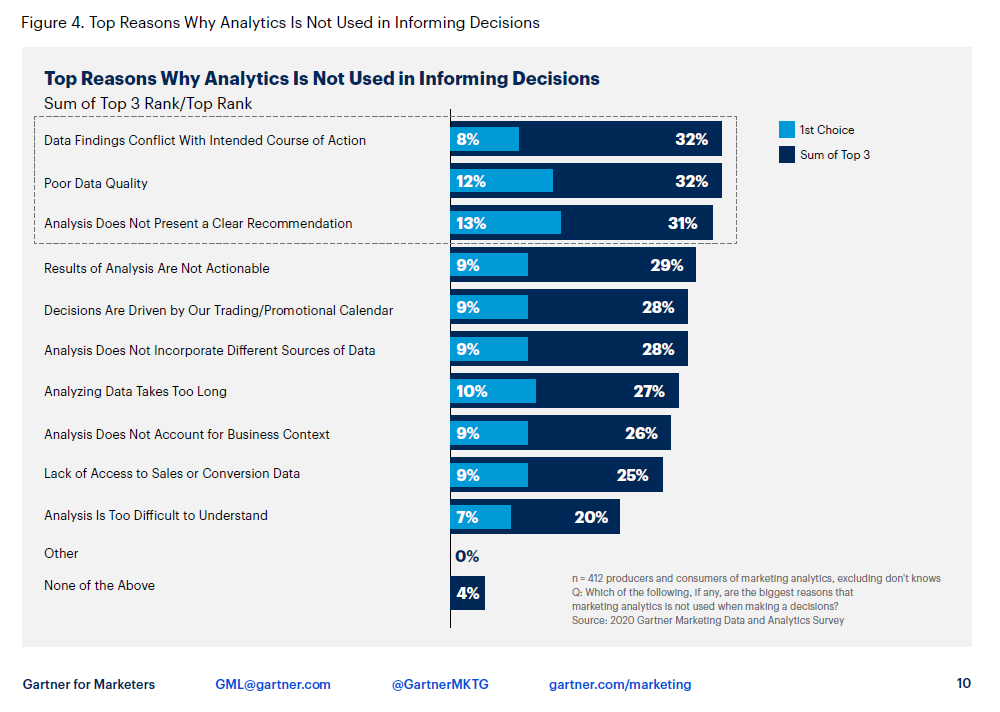
In the graphic above, several of the issues are related to matters of context, interpretation or presentation of analytics. These complaints are understandable when asking why analytics isn’t steering decision-making in the desired manner. However, I know from experience that a significant portion of the issues on the list can be eradicated – or significantly reduced – by deploying skilled data engineers at the right moments in analytics programmes.
Let’s take a closer look.
Poor Data Quality. For the reasons discussed above, I was not surprised to read that poor data quality was the joint-highest response from CMOs in this part of the survey. The important point for me here is that improving data quality is not a core competency for data analysts or data scientists. It is, however, one of the many things that data engineers are trained to do.
Analysis Does Not Incorporate Different Sources of Data. Consolidating data sources is another task that sits squarely in the data engineer’s skills sweet-spot. Analysts, of course, are experts at working out what data sources they need. Sometimes they can successfully combine data sources, although they typically must do so manually (and repeatedly). Analysts tend not to be trained in consolidating data sources, so asking them to do so often leads to slow, error-prone work, particularly when working with very large data sets.
Analysing Data Takes Too Long. Again, slow progress should be expected when analysts are navigating poor quality data or performing tasks that they are not trained for, such as manually consolidating data.
Lack of Access to Sales or Conversion Data. Fundamental data sources such as sales or conversion data are rarely excluded from successful marketing analytics. But if this data is too difficult or time-consuming to access for analysts, they may have no option but to do without. For CMOs with income- and budget-critical decisions to make, this is an unsatisfactory outcome. By contrast, deploy a data engineer to bring the data into a usable format, show them where the data lives, and they’ll have an automated data pipeline set-up in a matter of days or weeks. After that initial preparatory work, analysts will have ongoing access to the data in their analyses as and when they need it.
Analysts Are Struggling
Figure 10 of Gartner’s report shows the most common barriers facing marketing analytics departments (see graphic below). Once again, to my mind, two of the top four barriers to successful analytics are actually issues of data quality and availability – i.e. data engineering tasks – rather than the quality of the analysis itself.
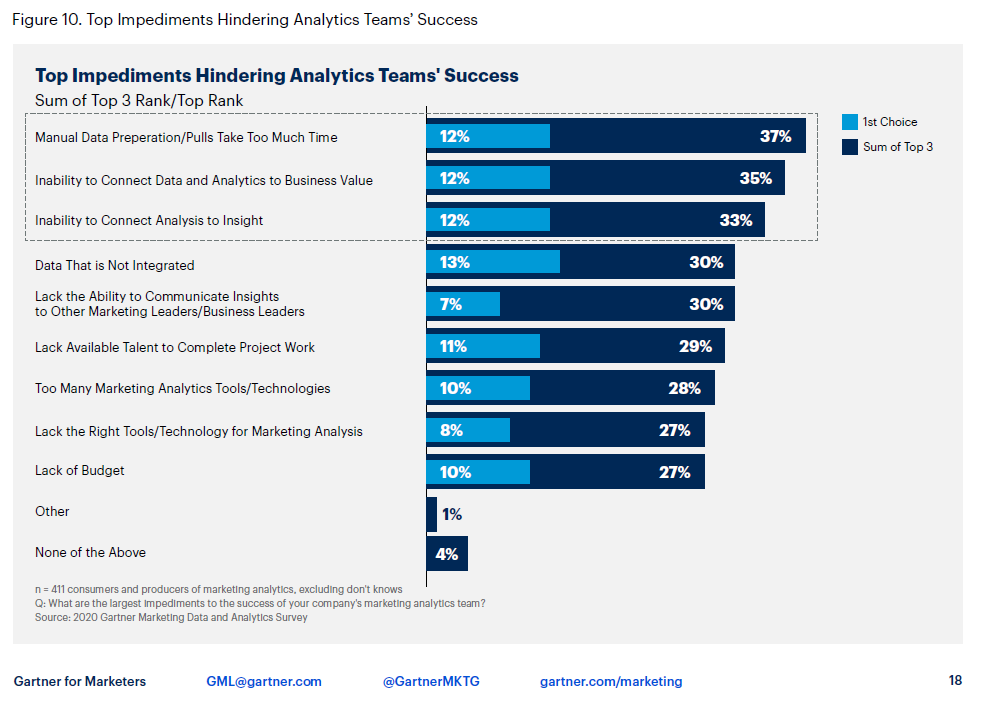
#1 “Manual data preparation / data pulls take too much time.” It seems clear to me that in the absence of well-engineered data, analysts have no choice but to manually extract and laboriously prepare data sets. When these data sets are extremely large, this can be a painfully slow (if not impossible) task for many analysts. Any time spent by analysts on manual data preparation and moving data from A to B is time taken away from extracting valuable insight. This burden can be dramatically reduced by data engineers, who are trained to format data appropriately and build automated pipelines that deliver clean, usable data directly to their analysts’ doorsteps.
#4 “Data That is Not Integrated.” Anyone else getting déjà vu?
Data Scientists’ Primary Activity: Data Management
Figure 12 in Gartner’s report presents the survey responses to the question “Which of the following activities is the marketing analytics team spending the most time on?”.
“Data Management, Data Integration and Data Formatting” is the most popular choice, garnering 64% of the votes. This appears to confirm that the majority of marketing analytics teams are spending the majority of their time on data engineering tasks.
While analytics and data science will always require some degree of data preparation, I find it disconcerting to see how disproportionately heavy the Data Management workload still is for marketing analytics teams. I expect most analysts and data scientists would rather the majority of their time be spent on activities that allowed them to do meaningful work that generates value.
And this statistic is not a one-off. Two years ago, in Gartner’s previous survey on the same topic, just over half of marketing analytics teams (52%) reported that they spend most of their time on data engineering work. That figure has now jumped to almost two-thirds (64%).
This is not entirely unexpected. Brands are dealing with more data sources and higher quantities of data than ever before. This trend shows no signs of slowing. And so, unless something changes, it’s safe to assume that the time analysts will need to spend on data management tasks will also continue to grow year-on-year.
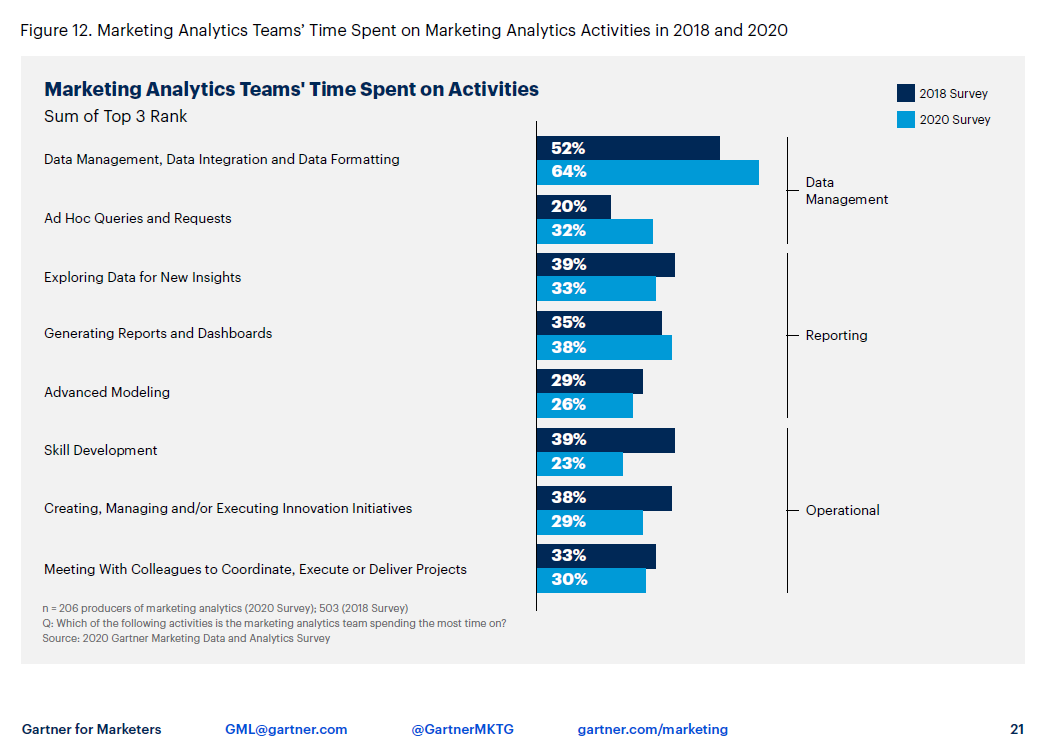
The fact that data management is the primary task of most marketing analytics teams – and significantly more so than exploring data for new insights – begs an obvious question: why is the enormous time investment in data management, data integration and data formatting required?
Does the data need such continuous hard work to maintain?
Not quite.
Taken together, the respondents’ answers appear to support the notion that it is because data analysts are also being expected to do the work of data engineers, poorly equipped as they may be to do so. In marketing teams where this is the case, the result is slow, inefficient analysis and sub-optimal utilisation of analysts and data scientists.
In Summary
Gartner’s report sheds valuable light on the situation facing many major enterprises. Significant investment has been – and continues to be – poured into building marketing analytics teams. The analytics teams were hired to extract insight from data that will guide decision-making.
The majority of Gartner’s survey respondents, however, report that marketing analytics teams are not making the desired impact.
One of the reasons for this dissatisfaction is painfully clear to me. Far too much of data analysts’ valuable time is being spent on data management tasks. Analyses take too long, slowed down by daily battles with poor quality data and repeated manual extracts. Under pressure to deliver, analysts can be forced to cut corners. They don’t utilise as many data sources as they would like. Their work delivers less actionable insight than the leadership demands. Confidence in the team wanes, and the pressure to deliver mounts further. The cycle repeats, and the pressure on CMOs to justify their continued investment grows.
It’s a vicious cycle that creates an unstable situation for marketing analytics teams and their CMOs.
Fortunately, the solution is equally clear. Bringing in data engineers to fix data quality issues or build automated data pipelines may not be as glamorous as launching a new AI or data science initiative. But without solid foundations to work from, poor quality data will continue to be a drain on analysts’ time and motivation.
By contrast, clean, connected data has a strong multiplier effect on analysts’ efficiency and impact.
Data engineers are the key skillset businesses require to make that shift.
In simple terms, CMOs must stop expecting their analysts to also do a data engineer’s job.
It may be sink or swim time for analysts. But keep in mind that even Michael Phelps might struggle with lead boots on.
Optima Connect are hands-on data solutions provider with advanced skills in data science and data engineering. Follow them on Twitter and LinkedIn.
Newsletter
Enjoy this? Get more.
Our monthly newsletter, The Edit, curates the very best of our latest content including articles, podcasts, video.
Become a member
Not a member yet?
Now it's time for you and your team to get involved. Get access to world-class events, exclusive publications, professional development, partner discounts and the chance to grow your network.